Introducing the New Apartment List Rent Estimate Methodology

Renters often start their housing search by determining what they can afford in the places they want to live. When property managers and landlords have a vacant unit to rent, they begin by learning what renters may be willing to pay for their property. Most successful interactions with the rental market necessitate an understanding of the market rent and how it is changing.
At Apartment List we track rents to help renters, owners, and the broader research community better understand how the rental market is evolving in real time. As an online platform, we have the ability to aggregate data from rental listings across the country to paint an accurate picture of how rents move both locally and nationally. There are many ways to make progress towards this goal and no single one-size-fits-all analysis to get there. To this end, we consistently strive to find ways of improving our methodology and promoting greater transparency in the process.
Today, Apartment List releases new rent data based on a new rent estimate methodology. This paper describes the objectives, mechanics, and improvements behind the new statistics. You may also access the full rent estimate dataset, as well as our National Rent Report and individual city reports that rely on these data.
Apartment List Rent Estimates
Not all rent statistics attempt to measure the same thing, so any discussion of a rent estimate methodology needs to start with the analytical goal. At Apartment List, we estimate the median rent across new leases signed in a given market and month. To capture how rents change in a market over time, we estimate the expected price change that a rental unit should experience if it were to be leased today.
We start with fully-representative median rent statistics for recent movers, estimated using the Census Bureau’s American Community Survey. We then extrapolate this data forward to the current month using a growth rate calculated from our listing data. We filter these data to capture the prices at which rental units transact, which quite often differ from initial or average list prices.1 Finally, we calculate growth rates using a same-unit analysis similar to Case-Shiller’s approach, comparing only units for which we observe transactions in multiple time periods to provide an accurate picture of rent growth that controls for compositional changes in the available inventory.
Before we dive into the details of the methodology, we want to outline a framework that underscores why we make the analytical decisions we do. Specifically, we designed our rent estimates with five criteria in mind. These goals continue to guide iterated improvements on our methodology to ensure that our statistics are as useful for our stakeholders as possible.
- Rent estimates reflect up-to-date data and current market conditions.
- Rent estimates reflect market forces and not composition shifts in what types of units are available in a given month.
- Rent estimates reflect prices paid by renters, not list prices for units that remain vacant.
- Rent estimates are representative of the entire rental market, and not the subset of properties that participate in our marketplace.
- Our methodology and models are transparent and accessible.
The Five Goals Behind Our Methodology
1. Rent estimates reflect up-to-date data and current market conditions.
As an online marketplace, Apartment List has access to real-time pricing and availability data that power our renter experience. Our first goal is to leverage this timeliness to get today’s estimates into the hands of our audience. In the final week of each month, we release data for approximately 500 cities, 50 states + DC, and the U.S. as a whole. This ensures that our estimates are locally-relevant and available without lag. Rents move quickly, and we aim to capture those movements as soon as they happen.
For historical rent data, the Census Bureau publishes the most comprehensive housing surveys in the nation. The most recent Census data (whose collection was unaffected by the COVID-19 pandemic) provides local median rent prices in 2019.2 Marrying this representative (yet somewhat outdated) Census data with real-time marketplace data allows us to deliver accurate and timely information on hundreds of markets across the nation.
2. Rent estimates reflect market forces and not composition shifts in what types of units are available in a given month.
Perhaps the simplest way to estimate rents in a city is to calculate the average price of all apartments available for rent each month. The problem with this approach is that the composition of any city’s for-rent inventory is constantly changing. So as averages shift, we don’t know if it is because landlords are actually changing rents or because different types of properties are hitting the market. A renter or property manager wondering if their unit is getting pricier won’t learn the answer from a simple “average of all listings” approach.
A quick example demonstrates this composition bias. Imagine a small housing market that quickly builds a large number of new luxury apartments. Now that the market composition includes many expensive listings, the average rent of available units has skyrocketed. But does that mean that a property manager in an older building can now charge more for their unit? And should their tenants expect to renew their lease at a much higher rent? More likely, the increase is simply driven by the fact that the composition of the market has changed.
To avoid this confusion we eschew simple means and medians. Instead, we capture repeat transactions - when a single apartment gets rented more than once over time - and check whether the transacted rent price has changed between those transactions.3 In our example above, the new luxury apartments have driven up average listing prices, but what if they also absorbed high-end demand in the market, allowing an older unit that once rented for $1,000 to now rent for $900? That—the repeat transaction—is what we capture and feed into our rent estimates.
3. Rent estimates reflect prices paid by renters, not list prices for units that remain vacant.
Relying on the average price of vacant units can introduce additional bias when there is a difference between an apartment’s list price (the price you see online) and its final transacted price (the price a renter agrees to pay when they sign a lease). Sometimes a vacant unit will be initially listed for more than local renters are willing to pay. In these cases the list price will eventually drop in an effort to entice renters, until it finally reaches a fair market price.
As shown in the charts below, these price drops follow temporal trends with important implications for rent estimates. Apartments rented in the same week that they are listed see almost no drop, but those that sit on the market for several months drop more than $50 in price, on average. Furthermore, the gap between list price and transacted price is greatest in fall and early winter, when rental demand is at its lowest.
Because list prices are systematically higher than transaction prices, a methodology based on average or median list prices will overstate the value of apartments in a given market. Furthermore, the seasonal component of this price differential can bias growth rates calculated from list prices. Our repeat-transaction approach solves for this bias by relying exclusively on transaction prices, thus filtering out the statistical noise generated by list price fluctuations.
4. Rent estimates are representative of the entire rental market, and not the subset of properties that participate in our marketplace.
We aim to estimate median rents for new leases across the entire market. However, online marketplaces (including ours) don’t provide a fully-representative sample of each market’s true housing inventory. Instead, they tend to skew towards newer and more-expensive apartments while underrepresenting older, cheaper units in lower-income neighborhoods. In turn, private listing data make markets appear more expensive than they really are.
The figures below demonstrate luxury bias in action in Washington DC. On the left, Census data show that a large concentration of DC’s rental units are located south of the Anacostia River. But on the right, we see how private listing sites underrepresent this part of the city. Instead, a higher concentration of apartment listings are found in pricier, northwestern neighborhoods.
We’ve extensively studied luxury bias in our own data to understand the scope of the problem and determine how to account for it. The issue is mitigated in our methodology by incorporating Census data. The American Community Survey is the gold-standard when it comes to capturing fully representative data that ecompasses the entire U.S. ACS rent estimates reflect all types of rental housing, from high-end luxury units to single-family rentals to public housing. The Census’s median rents are the starting point for our own estimates, which we then extrapolate forward to the present month using the growth rates calculated from Apartment List data.4
5. Our methodology and models are transparent and accessible.
Our rent estimates are designed to be a resource for everyone: renters, landlords, researchers, policymakers, and the general public. In the spirit of transparency, the technical details of our methodology are described below, as is a walkthrough example showing how we reach our final rent estimates in Los Angeles, CA. Furthermore, we provide answers to some common FAQs discussing the relative strengths and limitations of our methodology.
As researchers, we are constantly working to improve our analytic approach, so if you have any questions, suggestions, or critiques, don’t hesitate to send us a message at research@apartmentlist.com.
Methodology
We start with the universe of Apartment List property data, which consists of rental price and availability information from both verified partners and a number of listing feeds. These records power the real-time information on the Apartment List platform. We then apply two primary filters.
First, we identify each rental unit’s most-likely transaction price from its available time series of rents. As many renters know, rents for a particular apartment or home can change over the course of the unit’s availability on the market. Empirically, we find that rents tend to fall, on average, as a unit sits vacant. We estimate each unit’s transaction price by pinpointing the last available rental price before a unit ceases to be vacant.
Second, we filter our data down to matched series. These are units in our data that have plausibly been leased two or more times after appearing on our platform. The resulting data set is the rental market’s analog of the data powering a traditional repeat-sales index. To cleanse our estimated rent changes of composition effects, we build our estimates from price changes within the same unit being leased multiple times. This means that the units only appearing on our platform once do not affect our estimates. We filter these out.
Then, we use the final data set to estimate a rent index for each geography and month of interest. In order to let within-unit variation identify our rent index levels, we adopt a geometric repeat sales estimator, long used to calculate home price indices.5 In our context, we estimate
using OLS, where
The unit-level fixed effects estimate average rents per unit in the data and absorb the between-unit price variation in the data. The month-level fixed effects identify the average within-unit change in the natural logarithm of price, which is the goal of the index. We then convert these point estimates into predicted percent rent growth, using the fact that
The final step applies a three-month moving average to arrive at the final index.
Then, for additional context, we use our rent growth index to estimate median rents in each geography of interest. To make sure our estimates are as representative of the geography’s rental market as possible, we start with American Community Survey data on rents paid by recent movers and project these numbers forward to the present day using our rent growth index. At the time of this note, the most recent available ACS estimates are from the 2022 5-year survey.
We start with 2022 median rents for each geography of interest, extracted from Census table B25031 using the Census Bureau’s API system.6 These medians capture rents paid by long-time residents, so to move them closer to today’s fair market rents, we construct a recent mover adjustment (RMA), equal to the ratio of rents paid by recent movers to those paid by all renters. Recent movers are those who moved into their residence in 2021 or later, and their rents are extracted from Census table B25113. Each 2022 median rent is multiplied by the finest-available RMA (either corresponding to the city, county, CBSA, or state). With median rents paid by those signing new leases in hand, we project rents forward to the present month using the percent growth we estimated from the fixed-effects regression model.
To provide a real-world example of this methodology in action, below are the computational steps that estimate the median rent for one-bedroom apartments in Los Angeles, CA, in December 2023.
FAQs
Q: What makes your rent estimate methodology different from those of other rental platforms?
A: Other available rent estimates may be computed in different ways in order to accomplish different goals or to answer different questions. Many rent estimates are based on the list prices of vacant units currently available on listing sites, and thus do not account for the possible biases described above. Others are more sophisticated, using proprietary algorithms to predict rents. To our knowledge, though, our estimates are the only ones that address each of our outlined criteria.
Q: How do your rent estimates compare to other private listing sites?
A: For the reasons described above, other private listing sites tend to estimate higher rents than we do. Our final rent estimates can be interpreted as the median rent an apartment hunter could expect to find today, across their city’s entire market of rental options. Rent estimates derived from averages or medians taken over available listings are best interpreted as the average or median rent for the subset of vacant apartments that are listed on a given site in a given month.
Q: How do your rent estimates compare to census data?
A: While we provide lower estimates than other private listing platforms, ours tend to be higher than what you will find in the most recently-available Census data. This is because we project Census median rents forward in time, making up for the 1-2 year lag in Census data availability. In places where rents have risen during that time, our estimates will be higher. Moreover, we adjust the headline median rent figures reported by Census using a recent mover adjustment factor, which accounts for the fact that rents paid by recent movers tend to be higher than rents paid by all renters in a market, on average.
Q: Why are rents in some expensive core cities cheaper than those in surrounding suburbs?
A: This pattern can occur for a couple reasons. For one, the type of rental inventory available often differs from the core city to the suburbs. For example, in the core city, most two-bedroom units are apartments, while in the surrounding suburbs, the majority may be single-family homes with greater square footage and additional amenities (e.g., parking) that drive up the rental price. Second, suburbs tend to be smaller and more homogenous, making the distribution of rents more closely clustered around the median. By contrast, core cities tend to have a more diverse housing inventory, with pockets of expensive luxury units as well as many older, lower-rent buildings that pull the median downward.
Q: I have additional questions, how do I contact the Apartment List Research Team?
A: Simply shoot us an email: research@apartmentlist.com
Last updated: January 1, 2024
- This step represents a material departure from previous iterations of our rent estimate methodology, which were constructed using list prices.↩
- Census Table B25031: Median Gross Rent by Bedrooms. Newer 2020 tables are available, but we opt to use 2019 estimates out of concern for nonresponse bias in the 2020 estimates.↩
- This is similar to the Case-Shiller approach that has become industry-standard in housing economics.↩
- The resulting estimates are representative as long different types of rental inventory are not on systematically different rent growth trajectories, on average. While this parallel trends assumption is imperfect, we find it to be more plausible than the alternative assumption that rent levels on rental platforms are fully representative.↩
- The popular Case-Schiller approach for estimating a repeat sales index that we mention earlier is an arithmetic repeat sales estimator, which re-weights transactions of different prices to track changes in the total value of the for-sale housing stock. Our goal is to estimate the expected price change at the unit level, so our approach differs slightly.↩
- Specifically, via the censusapi R package.↩
Share this Article
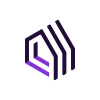